By Benjamin Lawson
Published: 7/7/2023
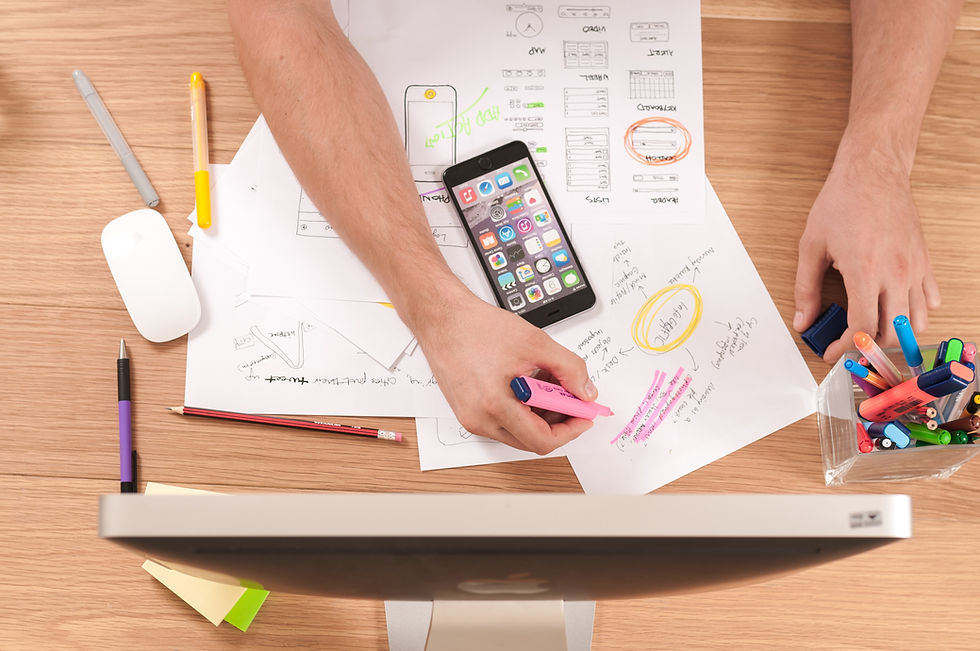
Introduction:
In today's data-driven world, organizations are increasingly recognizing the value of data science in gaining a competitive edge. Data science encompasses the application of scientific methods, processes, algorithms, and systems to extract knowledge and insights from structured and unstructured data. By harnessing the power of data science, businesses can make informed decisions, optimize processes, enhance customer experiences, and drive innovation. This article explores the transformative potential of data science and highlights key concepts, techniques, and real-world applications that are revolutionizing industries across the globe.
The Foundation of Data Science:
Data science relies on three fundamental pillars: statistics, programming, and domain expertise. Statistical knowledge allows data scientists to effectively analyze and interpret data, identify patterns, and make reliable predictions. Proficiency in programming languages such as Python or R enables them to manipulate and process large datasets efficiently. Moreover, domain expertise empowers data scientists to ask the right questions, understand business needs, and derive actionable insights from data.
Data Collection and Preparation:
The success of any data science project hinges on the quality and relevance of the data. Data collection involves gathering data from various sources, such as databases, APIs, sensors, social media platforms, and more. Data scientists must then preprocess and clean the data, addressing issues like missing values, outliers, and inconsistencies. This stage also involves data integration, transformation, and feature engineering, where data is prepared in a format suitable for analysis.
Exploratory Data Analysis (EDA):
EDA serves as the initial step in extracting insights from data. Data scientists employ statistical techniques and data visualization tools to uncover patterns, relationships, and anomalies in the dataset. EDA aids in formulating hypotheses, identifying potential challenges, and directing subsequent analysis. By exploring the data visually, data scientists can communicate their findings effectively to stakeholders and gain a deeper understanding of the problem at hand.
Machine Learning and Predictive Analytics:
Machine learning algorithms lie at the heart of data science, enabling the automation of analytical model building. By training models on historical data, data scientists can develop predictive and prescriptive models to make accurate forecasts and optimize decision-making. Supervised learning techniques, such as regression and classification, learn from labeled data, while unsupervised learning algorithms, like clustering and dimensionality reduction, uncover hidden patterns in unlabeled data.
Deep Learning and Neural Networks:
Deep learning, a subset of machine learning, focuses on artificial neural networks that mimic the human brain's structure and function. Deep neural networks excel in processing unstructured data, such as images, audio, and text. They have revolutionized fields like computer vision, natural language processing, and speech recognition. Deep learning algorithms, such as convolutional neural networks (CNNs) and recurrent neural networks (RNNs), have achieved remarkable breakthroughs in image recognition, autonomous driving, and language translation.
Data Science in Action: Real-World Applications:
Data science is making a significant impact across various industries. In healthcare, it aids in disease prediction, drug discovery, and personalized medicine. E-commerce companies leverage data science for customer segmentation, recommendation systems, and fraud detection. Transportation and logistics industries optimize routes, minimize fuel consumption, and enhance supply chain management through data science. Financial institutions utilize data science for risk analysis, fraud prevention, and algorithmic trading.
Ethical Considerations and Privacy:
As data science becomes increasingly pervasive, ethical considerations and privacy concerns come to the forefront. Responsible data collection, data anonymization, and ensuring data security are crucial for maintaining public trust. Data scientists must adhere to ethical guidelines, comply with data protection regulations, and address potential biases and discrimination inherent in algorithms.
Conclusion:
Data science has emerged as a game-changer, revolutionizing how organizations derive insights and make data-driven decisions. From statistical analysis to advanced machine learning and deep learning techniques, data science enables businesses to unlock the full potential of their data. By leveraging data science effectively and responsibly, organizations can gain a competitive advantage, drive innovation, and pave the way for a data-driven future.
Comments