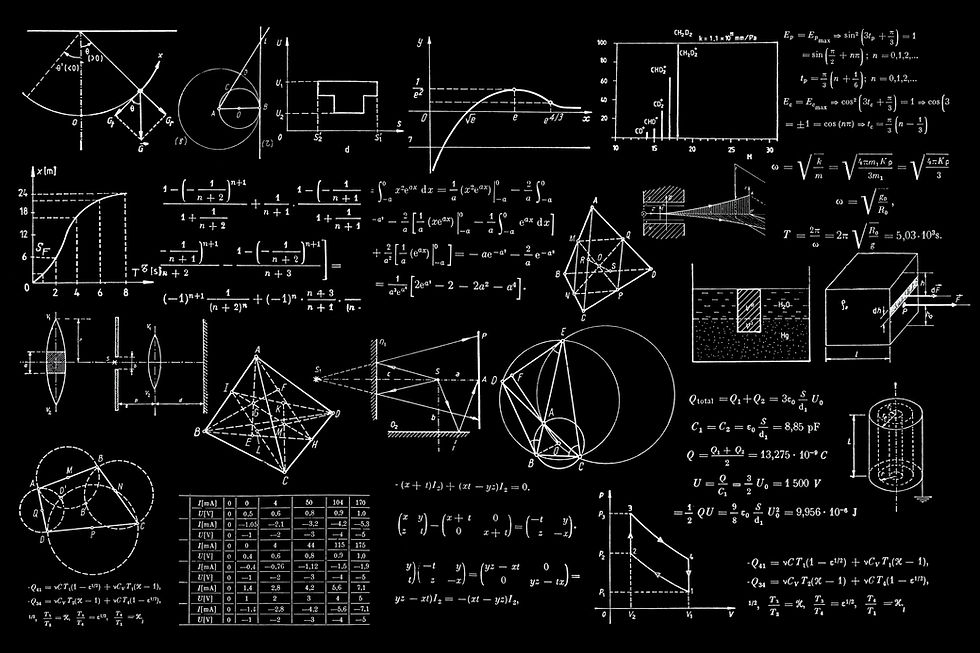
The worlds of data-driven machine learning and physics-based modeling have historically been viewed as separate domains. However, the emerging field of Physics-Informed Neural Networks (PINNs) is breaking down these traditional boundaries by integrating the strengths of both approaches. By infusing physics principles into neural networks, PINNs offer a promising solution to challenges where data is scarce, noisy, or expensive to collect. In this article, we delve into the concept of Physics-Informed Neural Networks, exploring their applications, benefits, and the unique synergy they bring to the table.
The Need for Physics-Informed Approaches in Machine Learning:
While traditional machine learning techniques excel at processing large volumes of data, they can struggle when faced with situations where physical laws and constraints are important. PINNs address this limitation by incorporating domain-specific physics knowledge directly into neural networks, enabling them to generalize more effectively, even with limited data.
Blending Neural Networks with Physics Insights:
Physics-Informed Neural Networks combine the flexibility of neural networks with the laws of physics governing a problem. This integration allows the network to learn from both data and underlying physical principles, making it capable of accurate predictions and simulations while maintaining physical consistency.
The Architecture of PINNs:
In a PINN architecture, neural networks are augmented with additional terms representing physical laws and constraints. These terms act as regularizers, guiding the network to conform to known physics. By solving the governing equations alongside data-driven optimization, PINNs provide robust and accurate predictions.
Solving Inverse Problems and Data-Scarce Situations:
Inverse problems, where the goal is to deduce inputs from outputs, often suffer from ill-posedness and lack of data. PINNs excel in these scenarios, leveraging physical insights to regularize the solution space and generate accurate predictions even with limited observations.
Applications of PINNs:
Physics-Informed Neural Networks find applications in a variety of fields. In fluid dynamics, PINNs aid in simulating complex flow behaviors with sparse data. In medical imaging, they enhance image reconstruction from limited measurements. Similarly, they assist in designing materials with specific properties by bridging experimental data and physical models.
Benefits of Physics-Informed Neural Networks:
PINNs offer several advantages, including reduced sensitivity to noisy data, better generalization with limited observations, and the ability to handle complex physical constraints. Their ability to seamlessly incorporate domain knowledge enhances the interpretability and reliability of the predictions.
Training and Challenges:
Training PINNs requires a careful balance between data-driven optimization and adherence to physics laws. Challenges include determining appropriate regularization terms, handling uncertain physics equations, and effectively utilizing hybrid datasets comprising both observational and physical data.
Towards the Future of PINNs:
As the field of Physics-Informed Neural Networks matures, researchers are exploring hybrid models that combine traditional physics-based simulations with neural networks. Additionally, efforts are being made to automate the discovery of governing equations from data, expanding the applicability of PINNs to a wider range of problems.
Ethical and Practical Considerations:
While PINNs offer exciting possibilities, their deployment should be guided by ethical considerations. Ensuring the accuracy of the integrated physics and maintaining transparency in model predictions are essential for building trust in applications that impact critical decision-making.
Physics-Informed Neural Networks represent a groundbreaking convergence of data science and physics, providing a bridge between the strengths of both disciplines. By fusing domain-specific knowledge with the adaptability of neural networks, PINNs unlock new avenues for accurate predictions in scenarios where data is limited, noisy, or incomplete. As this field continues to evolve, the potential applications and impact of Physics-Informed Neural Networks are boundless, ushering in a new era of intelligent systems that respect and harness the laws of the physical world.
Comments