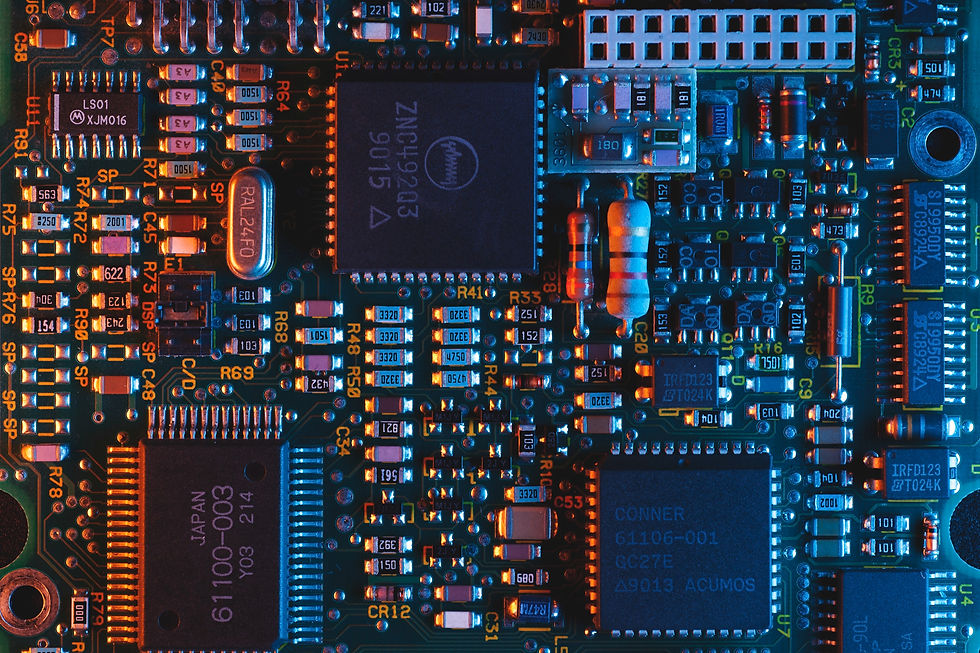
Quantum computing, an emerging field at the intersection of quantum mechanics and computer science, holds the promise of revolutionizing various industries by solving complex problems that are beyond the capabilities of classical computers. In the realm of data science, quantum computing is poised to bring transformative changes, offering the potential to accelerate data analysis, optimize algorithms, and solve computational challenges that were once thought to be insurmountable. In this article, we delve into the role of quantum computing in data science and its implications for the future of data analysis.
Quantum Bits (Qubits) and Their Potential:
At the heart of quantum computing are qubits, the quantum counterparts of classical bits. Unlike classical bits, qubits can exist in a superposition of states, enabling them to represent multiple values simultaneously. This property allows quantum computers to process vast amounts of information in parallel, making them potentially more powerful for certain tasks compared to classical computers. Data scientists are exploring how qubits can be harnessed to perform complex computations and analyses in a fraction of the time required by classical methods.
Quantum Algorithms for Data Analysis:
Quantum computing introduces novel algorithms that can transform the way data is analyzed. One such algorithm is the Quantum Principal Component Analysis (QPCA), which can efficiently extract the most significant patterns from large datasets. Another example is the Quantum Support Vector Machine (QSVM), which holds promise for solving complex classification problems in machine learning. These quantum algorithms have the potential to significantly speed up data analysis and open new avenues for discovering insights from data.
Optimization and Quantum Annealing:
Optimization problems, which involve finding the best solution from a large set of possibilities, are prevalent in data science. Quantum annealing is a technique that leverages quantum principles to solve optimization problems efficiently. It has the potential to revolutionize areas such as portfolio optimization, supply chain management, and network optimization. Quantum annealers, such as those developed by D-Wave Systems, are being explored to address complex optimization challenges in data-driven decision-making.
Quantum Machine Learning:
Quantum computing also intersects with machine learning, giving rise to the field of quantum machine learning (QML). QML explores how quantum computers can enhance classical machine learning algorithms and enable the discovery of new patterns in data. Quantum neural networks and quantum-enhanced clustering algorithms are examples of how quantum computing can be applied to machine learning tasks, potentially leading to breakthroughs in pattern recognition and data-driven predictions.
Challenges and Considerations:
While the potential of quantum computing in data science is exciting, there are challenges to overcome. Quantum computers are sensitive to noise and require sophisticated error correction techniques to maintain the integrity of computations. Additionally, quantum computers are not a one-size-fits-all solution; they excel in certain types of problems but may not provide a significant advantage in others. Data scientists must carefully assess which problems are amenable to quantum computing's strengths and limitations.
The Path Forward:
As quantum computing technology advances, collaboration between quantum physicists, computer scientists, and data analysts becomes crucial. Hybrid approaches that combine classical and quantum computing techniques are being explored to leverage the strengths of both paradigms. Quantum simulators, which simulate quantum behavior on classical computers, also play a role in understanding and optimizing quantum algorithms.
Quantum computing is poised to transform the landscape of data science by providing unprecedented computational power and new ways of approaching complex problems. While practical quantum computers capable of outperforming classical counterparts are still in their infancy, rapid progress is being made. The marriage of quantum computing and data science holds the potential to unlock new insights, accelerate data analysis, and reshape the way we process and understand information. As researchers continue to push the boundaries of quantum computing, its role in data science is likely to evolve, ushering in a new era of computational possibilities.
Comments